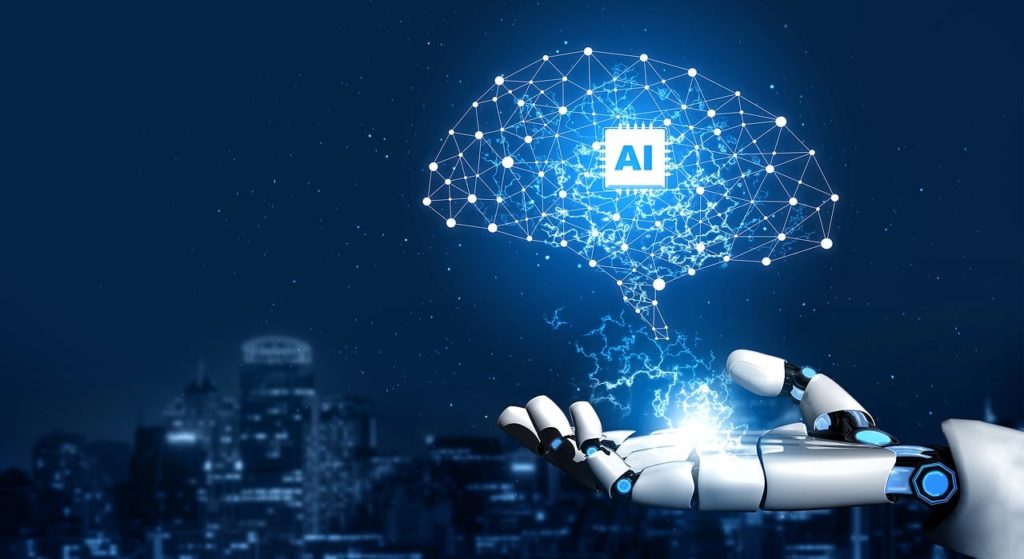
Researchers are releasing the main dataset from an ambitious study exploring the biomarkers and environmental factors that may influence the development of type 2 diabetes. This study involves participants with no diabetes and those at various stages of the condition. The initial findings suggest a rich and unique set of information that differs from previous research.
Data from customized environmental sensors installed in participants’ homes reveal a clear link between disease states and exposure to fine particulate pollution. The collected information also includes survey responses, depression scale scores, eye imaging scans, traditional glucose measurements, and various other biological variables.
These data are intended to be mined by artificial intelligence for novel insights about risks, preventive measures, and pathways between disease and health.
“We observe evidence of diversity among patients with type 2 diabetes, indicating that their experiences and challenges are not uniform. With access to increasingly large and detailed datasets, researchers will have the opportunity to explore these differences in depth,” stated Dr. Cecilia Lee, a professor of ophthalmology at the University of Washington School of Medicine.
She expressed excitement at the quality of the collected data, representing 1,067 people, just 25% of the study’s total expected enrollees.
Lee is the program director of AI-READI (Artificial Intelligence Ready and Equitable Atlas for Diabetes Insights), a National Institutes of Health-supported initiative that aims to collect and share AI-ready data for global scientists to analyze for new clues about health and disease.
The authors restated their aim to gather health information from a more racially and ethnically diverse population than previously measured, and to make the resulting data ready, technically and ethically, for AI mining.
“This discovery process has been invigorating,” said Dr. Aaron Lee, a UW Medicine professor of ophthalmology and the project’s principal investigator. “We’re a consortium of seven institutions and multidisciplinary teams that had never worked together. But we have shared goals of drawing on unbiased data and protecting the security of that data as we make it accessible to colleagues everywhere.”
At study sites in Seattle, San Diego, and Birmingham, Alabama, recruiters are collectively enrolling 4,000 participants, with inclusion criteria promoting balance:
- race/ethnicity (1,000 each – white, Black, Hispanic and Asian)
- disease severity (1,000 each – no diabetes, prediabetes, medication/non-insulin-controlled and insulin-controlled type 2 diabetes)
- sex (equal male/female split)
“Conventionally, scientists are examining pathogenesis — how people become diseased — and risk factors,” Aaron Lee said. “We want our datasets also to be studied for salutogenesis, or factors that contribute to health. So if your diabetes improves, what factors might contribute to that? We expect that the flagship dataset will lead to novel discoveries about type 2 diabetes in both of these ways.”
He added that by collecting more deeply characterizing data from many people, the researchers hope to create pseudo health histories of how a person might progress from disease to full health and from full health to disease.
The data are hosted on a custom online platform and produced in two sets: a controlled-access set requiring a usage agreement and a registered, publicly available version stripped of HIPAA-protected information.