A machine-learning tool developed by investigators at Weill Cornell Medicine and Hospital for Special Surgery (HSS) can differentiate between subtypes of rheumatoid arthritis (RA), potentially improving care for the complex condition.
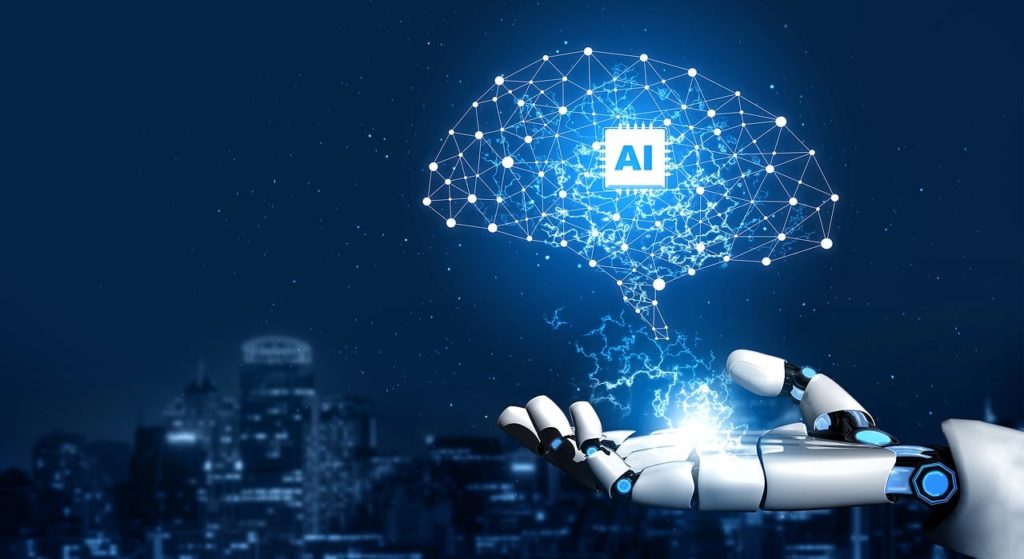
The study, published on August 29 in Nature Communications, demonstrates that artificial intelligence and machine learning technologies can accurately and swiftly subtype pathology samples from patients with RA.
“Our tool automates the analysis of pathology slides, which may one day lead to more precise and efficient disease diagnosis and personalized treatment for RA,” said Dr. Fei Wang, a professor of population health sciences and the founding director of the Institute of AI for Digital Health (AIDH) in the Department of Population Health Sciences at Weill Cornell Medicine. “It shows that machine learning can potentially transform pathological assessment of many diseases.”
Several machine-learning tools are being developed for the automatic analysis of pathology slides in oncology. Dr Wang and his colleagues are expanding the use of this technology in other clinical specialities.
Automating a Slow Process
In the most recent study, Dr. Wang collaborated with Dr. Richard Bell and Dr. Lionel Ivashkiv to automate the process of categorizing RA tissue samples into three subtypes. This may assist clinicians in selecting the most effective therapy for individual patients.
Pathologists currently manually classify arthritis subtypes using a rubric to identify cell and tissue characteristics in biopsy samples from human patients. This process is slow, adds to the cost of research, and may lead to inconsistencies between pathologists.
“It’s the analytical bottleneck of pathology research,” Dr. Bell said. “It is very time-consuming and tedious.”
The team initially trained its algorithm on rheumatoid arthritis (RA) samples from one group of mice, refining its capability to differentiate tissue and cell types in the sample and categorize them by subtype. They verified the tool’s effectiveness using a separate set of samples. The tool provided new findings regarding the impact of treatments on the mice, showing reduced cartilage degradation within six weeks of administering commonly used RA treatments.
They then used the tool on patient biopsy samples from the Accelerating Medicines Partnership Rheumatoid Arthritis research consortium and demonstrated its ability to accurately and quickly analyze human clinical samples. The researchers are currently validating the tool with more patient samples and figuring out the most effective way to integrate this new tool into pathologists’ workflows.
A Step Toward Personalized Medicine
“It’s the first step toward more personalized RA care,” Dr. Bell said. “If you can build an algorithm that identifies a patient’s subtype, you’ll be able to get patients the treatments they need more quickly.”
The technology could offer new insights into the disease by identifying unexpected tissue changes that humans might overlook. By reducing the time it takes for pathologists to subtype, the tool may also lower costs and improve the effectiveness of clinical trials for testing treatments on patients with different RA subtypes.
“By integrating pathology slides with clinical information, this tool demonstrates AI’s growing impact in advancing personalized medicine,” said Dr. Rainu Kaushal, senior associate dean for clinical research and chair of the Department of Population Health Sciences at Weill Cornell Medicine. “This research is particularly exciting as it opens new pathways for detection and treatment, making significant strides in how we understand and care for people with rheumatoid arthritis.”
The team is working on developing similar tools to evaluate osteoarthritis, disc degeneration, and tendinopathy. Additionally, Dr. Wang’s team is looking into defining disease subtypes using broader biomedical information. For instance, they have recently shown that machine learning can differentiate three subtypes of Parkinson’s disease. “We hope that our research will encourage more computational research in developing machine learning tools for a wider range of diseases,” said Dr. Wang.
“This work represents a significant advancement in analyzing RA tissues that can be applied to benefit patients,” stated Dr. Ivashkiv.